November 9, 2023 | 3 Min
Six Security Threats to Watch Out for with Generative AI
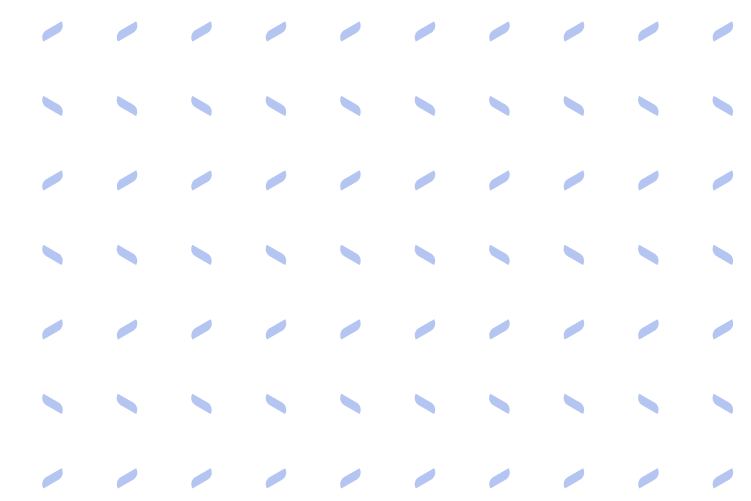
With AI technology rapidly changing how businesses operate, an exploration of new security threats has become critically important. As a Senior Cybersecurity Specialist, I wanted to share what we’re looking at here at Amplience and what our customers should consider when evaluating AI solutions.
Let’s take a closer look at some of the threats I’ve identified and some of my key recommendations for protecting your business.
1. Generative AI can enhance phishing opportunities
Generative AI could not only create more convincing phishing emails, but it also allows for the generation of images and videos that could aid credibility in a phishing attempt. Forged documents, pictures of fake events and even video or audio clips from a top executive are now only a few clicks away for bad actors. And any of these can be more convincing to a potential target.
I highly recommend you educate your employees, so they know what to look out for in phishing emails, as well as in AI-generated images, to help them identify anomalies such as visual tearing, for example.
2. Employees can leak confidential information to open AI systems
As generative AI tools gain popularity, more and more companies are seeing their confidential materials being leaked into open source AI systems. Humans have become trusting of the intelligence behind AI systems such as ChatGPT and readily share secrets or sensitive information with it. Many generative AI systems also operate on a feedback loop where inputs from users are fed into the next set of training data, leading to confidential material from company A potentially being available to malicious actors.
I recommend that confidentiality and information security training for employees includes awareness of this new threat. Your employees should view generative AI with the same healthy level of mistrust as other publicly accessible areas of the internet.
3. Powerful machine learning increases the need for tight security controls
The machine learning functionality used in the development, and often deployment, of generative AI requires powerful machines. These machines come at a higher cost than typical servers, and as such their overuse through negligence, repeated querying from external sources, or malware such as bitcoin miners, can become extremely costly.
Machines should be turned off or asleep when not in active use, and developers should be encouraged to call out any forgotten or abandoned servers for triage and termination.
Monitoring should be enabled on machines for the detection of malware, and special focus should be made on the detection of calls out to known cryptocurrency domains or addresses.
4. Malicious actors can poison training data
Generative AI in particular needs a large amount of training data, but malicious actors are developing new and subtle techniques to tamper with that data and create negative outcomes. A recent paper showed, with certain techniques, two standard, large datasets could be poisoned for less than $100. With this kind of attack, a self-driving car can be manipulated into ignoring stop signs, and generative AI into creating offensive media.
Adversarial and drift detection capabilities must be developed in order to filter out bad data. At Amplience, we use drift detection to help with the data sanitization process, speeding up our analysis.
5. Machine learning can increase the need to stay on top of technical debt
Often, the machine learning systems that power generative AI can dwarf the support systems that support them, such as data collection, resource management, and verification. This means that special effort must be made to address technical debt at a systems level.
Improving collaboration and communication between architects and the resources that support them will be critical to mitigate the risk of technical debt.
6. Generative AI has no basic understanding of bias and fairness
Generative AI has no inherent understanding of morality, and so, unless built otherwise, will offer unfiltered content. This can not only have a reputational impact, but also be detrimental to the usefulness of its outputs. A text generative AI that has been taught to frequently use foul language cannot be helpful in producing marketing content, and an image GenAI heavily biased towards light skin tones is practically unusable for a beauty line developed for dark skin.
Unbiased and fair inputs must be carefully cultivated to avoid pollution of your generative AI data. And the governments of both the UK and US agree, both have made it clear that algorithmic biases can be considered discriminatory where decision making is involved, under similar circumstances to other forms of discrimination, and that it is important for algorithms to be used in a way that is fair and seen to be fair.
Here at Amplience, from the very beginning of the AI conversation, we have been considering fairness and the elimination of bias. Every employee has a role to play in this process and as such, we encourage healthy discussion on this topic. In the security team, we take these considerations further, developing cutting-edge methods to include them in a more secure AI development pipeline.
In conclusion
While there are novel risks to look out for in the rapidly evolving world of generative AI, our team here at Amplience will continue to monitor for and adjust processes around novel and existing threats. Please contact us anytime to learn more about our approach.